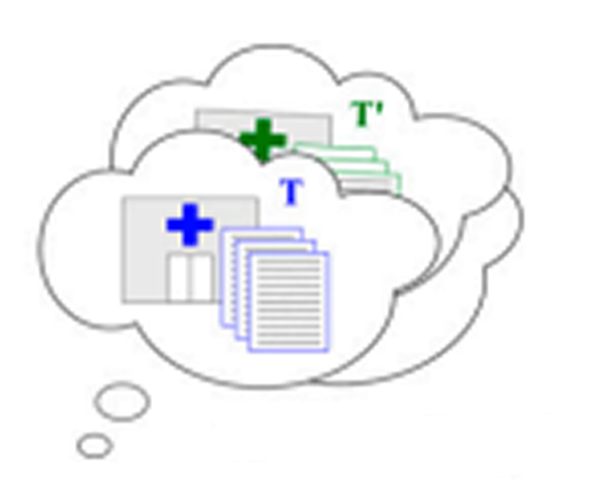
A Panel Discussion with Q&A
Tuesday, March 8, 2022
12:00 pm – 1:00 pm EST
The slides can be found here.
Presenters:
Christoph Kern, Ph.D.
University of Mannheim (Postdoc)
University of Maryland (Research Assistant Professor)
Michael P. Kim
Miller Institute at UC Berkeley (Postdoc)
Moderator:
Frauke Kreuter, Ph.D.
Universal adaptability: Target-independent inference that competes with propensity scoring
Authors: Michael P. Kim, Christoph Kern, Shafi Goldwasser, Frauke Kreuter, and Omer Reingold
The gold-standard approaches for gleaning statistically valid conclusions from data involve random sampling from the population. Collecting properly randomized data, however, can be challenging, so modern statistical methods, including propensity score reweighting, aim to enable valid inferences when random sampling is not feasible. We put forth an approach for making inferences based on available data from a source population that may differ in composition in unknown ways from an eventual target population. Whereas propensity scoring requires a separate estimation procedure for each different target population, we show how to build a single estimator, based on source data alone, that allows for efficient and accurate estimates on any downstream target data. We demonstrate, theoretically and empirically, that our target-independent approach to inference, which we dub “universal adaptability,” is competitive with target-specific approaches that rely on propensity scoring. Our approach builds on a surprising connection between the problem of inferences in unspecified target populations and the multicalibration problem, studied in the burgeoning field of algorithmic fairness. We show how the multicalibration framework can be employed to yield valid inferences from a single source population across a diverse set of target populations.
The SoDa Center at UMD
The powerful information available in large social science data sets is critical to understanding and addressing many of our nation and world’s most pressing challenges: from Covid-19 to racial, social and economic injustice; and from climate change to deep and damaging political and cultural divides. To help address these challenges, the University of Maryland has launched a new Social Data Science Center (SoDa) designed to advance research, education, and applications of social data measurement and analysis. This center leverages UMD’s strengths in survey methods, measurement, information management, visualization, and analytics. Facebook is providing support for the center’s research and education programs over the next three years.